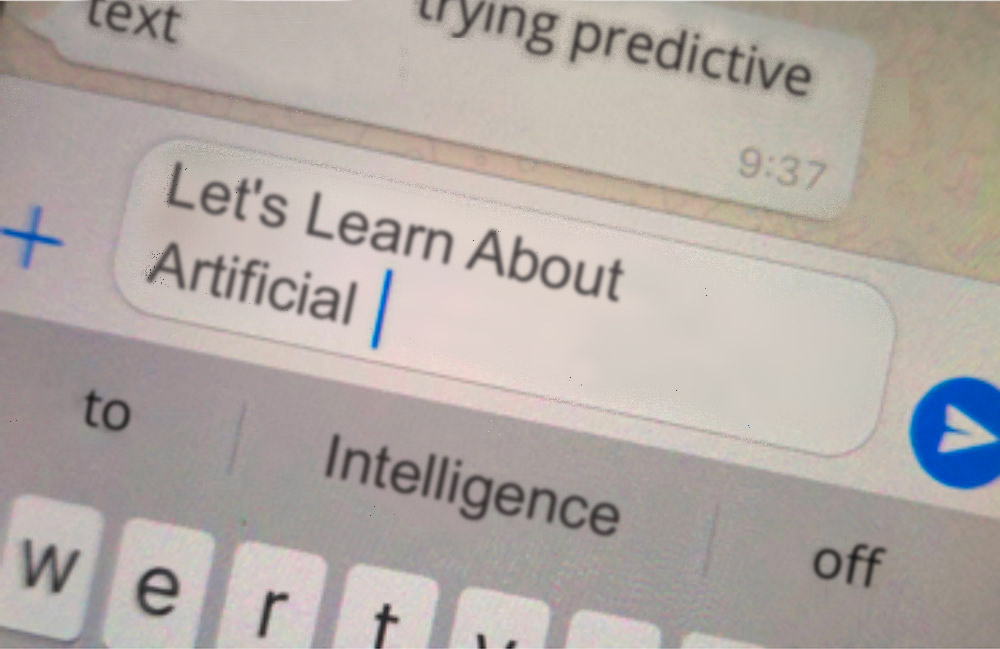
Artificial Intelligence (AI) is probably one of the fastest growing branches of computer science today. Despite emerging in its own right more than 70 years ago, AI is currently generating the greatest interest in its history because of the revolution it is bringing about in today’s market. For this reason, and because Artificial Intelligence is raising many questions and much interest among our customers, Xeridia will be publishing a series of articles on our blog, beginning with this introduction that aims to put the concept of Artificial Intelligence into context.
Until recently, computing power was limited, which meant that Artificial Intelligence delivered very poor results for those problems to which it was applied. This led to several periods of discontent in the industry historically, and to a considerable reduction in both the interest in this discipline and the number of researchers dedicated to it.
However, in recent years, Artificial Intelligence has been gaining significant momentum thanks to its ability to use computers to solve problems that were not previously considered possible, at levels that had never before been achieved. Even mobile devices have benefited from research in this field, for example through the predictive text feature of the keyboard, unlocking the screen with a fingerprint, or facial recognition in images captured by the camera. Several reasons could be listed as providing the driving force behind AI. However, the democratisation of computing power stands out in particular, especially after the publication in 2009 of the first scientific article on the large-scale parallelisation of AI computing using GPUs[1] and of another in 2010[2] demonstrating its use in the automatic recognition of handwritten digits, outperforming human capabilities in this task for the first time. Thus, Artificial Intelligence takes its name from the ability of an electronic device to solve problems for which intelligence is required and which could not be solved using a computer in a traditional way (through programming).
Looking back in history, Artificial Intelligence was born more than 70 years ago into the hands of Alan Turing, considered the father of this discipline. In 1936, he devised a computational model consisting of a tape head on an infinitely long tape that can read from it, write to it and move along it. This computational model was called a Turing machine and can represent any computational operation, in other words, any computation can be reduced and represented using a Turing machine. This raised the following question: If a brain performs computational operations, could it be reduced and represented using a Turing machine? If this were possible, it would mean that operations that we consider intelligent, such as detecting objects in images, classifying sounds or even the very functioning of a brain’s consciousness, could be automated. We cannot say for sure that the latter will ever happen. However, there have been advances in simulating or approximating intelligent behaviours, which is why subfields of Artificial Intelligence have emerged, such as Computer Vision (CV), Machine Learning (ML) and Natural Language Processing (NLP). In the posts that we will be publishing on this subject, we will gradually delve into each subfield with the aim of demonstrating that Artificial Intelligence is beginning to show the level of maturity required to be used to solve problems that, until just a few years ago, were considered unsolvable by machines.
Curiously, there is a strong relationship between neuroscience and Artificial Intelligence, with contributions in one field even serving as the basis for contributions in the other. One of the most striking cases is that of Geoffrey Hinton, considered the godfather of Deep Learning, a sub-branch of Automatic Learning that we will discuss in a future blogpost. A scientist, Hinton studied and dedicated much of his life to cognitive psychology while maintaining a focus on computer science, gaining a PhD in Artificial Intelligence. This allowed him to propose ideas used in cognitive psychology as a basis for Artificial Intelligence algorithms, becoming one of the world’s leading players in the field of AI. Combining both disciplines into knowledge means that they can be studied from different points of view: neuroscience through the reverse engineering of what already exists and Artificial Intelligence through the creation of algorithms based on ignorance. It is possible that, in the future, both disciplines will converge enough to become one.
Keep an eye on the Xeridia blog so you don’t miss any of our articles on Artificial Intelligence. You can subscribe to our newsletter or follow us on Twitter or LinkedIn.
Iván de Paz Centeno is a Data Scientist and R&D Engineer at Xeridia
[1] Raina, R., Madhavan, A., & Ng, A. Y. (2009, June). Large-scale Deep Unsupervised Learning using Graphics Processors. In Proceedings of the 26th Annual International Conference on Machine Learning (pp. 873-880). ACM.
[2] Cireşan, D. C., Meier, U., Gambardella, L. M., & Schmidhuber, J. (2010). Deep, Big, Simple Neural Nets for Handwritten Digit Recognition. Neural Computation, 22(12), 3207-3220.